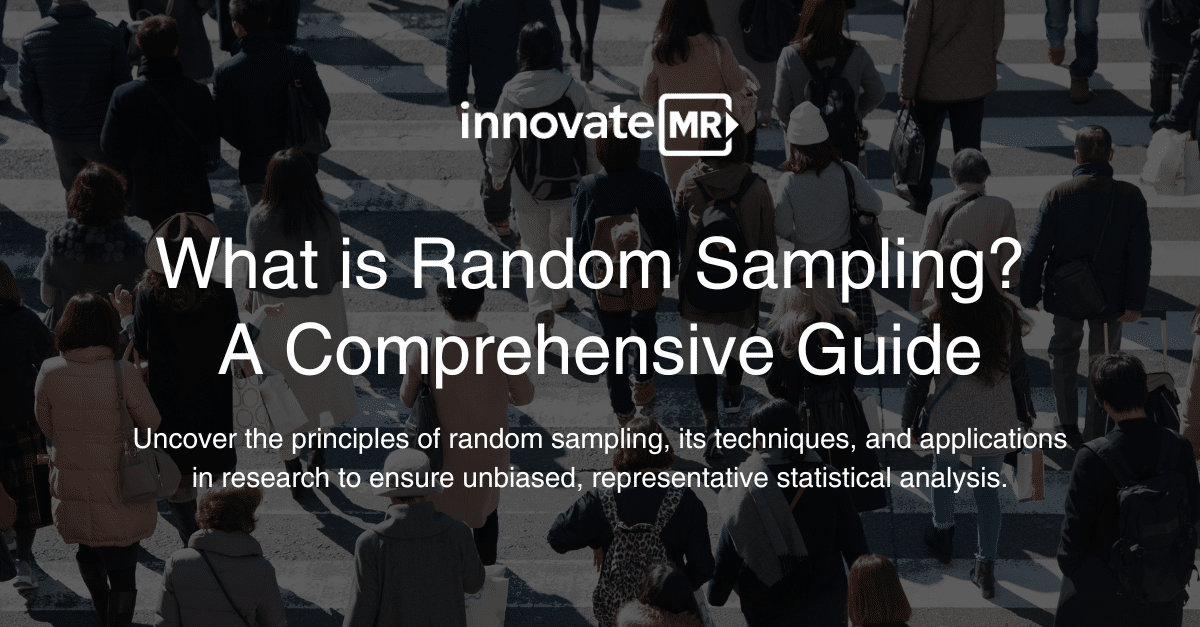
Sampling methods serve as the cornerstone of statistical inference, aiding researchers in making informed decisions about populations based on samples. Amidst various sampling techniques, such as snowball sampling and audience sampling. random sampling holds a significant position owing to its capability to provide unbiased and representative subsets of populations. Delving deeper into this technique sheds light on its essence and indispensable role in statistical analysis.
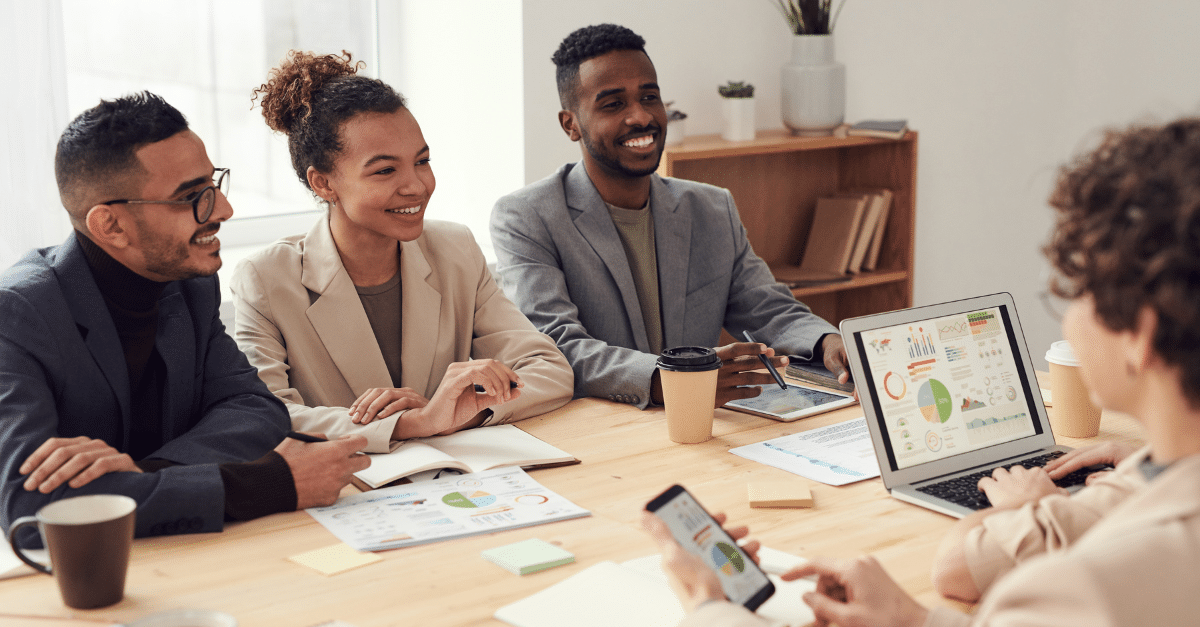
What Constitutes Random Sampling?
Random sampling involves the unbiased selection of individuals or items from a larger population in such a manner that each member has an equal probability of being chosen. This approach ensures that the selected sample mirrors the characteristics of the entire population, thereby allowing researchers to make reliable generalizations and predictions.
The Significance of Random Sampling
The fundamental importance of random sampling lies in its ability to minimize biases inherent in other sampling methods. Ensuring that each element in the population has an equal chance of being selected promotes the creation of samples that accurately reflect the population’s characteristics. Consequently, this technique serves as a cornerstone for various research studies, surveys, and experiments across diverse domains.
Exploring Techniques of Random Sampling
Simple Random Sampling
This method involves randomly selecting elements from the population without any specific pattern or order. It ensures each member of the population has an equal chance of being included in the sample, often facilitated by random number generators or drawing lots.
Systematic Sampling
In systematic sampling, every nth element from the population is chosen after an initial random start. This method provides an organized way of sampling and is particularly useful when the population is ordered in a sequence.
Stratified Sampling
Stratified sampling involves dividing the population into homogeneous subgroups or strata based on certain characteristics. Samples are then randomly selected from each stratum, ensuring representation from different segments of the population.
Cluster Sampling
Cluster sampling involves dividing the population into clusters or groups, usually based on geographical location or other grouping factors. Researchers then randomly select entire clusters as samples, making this method particularly practical when it’s challenging to enumerate or access individual elements.
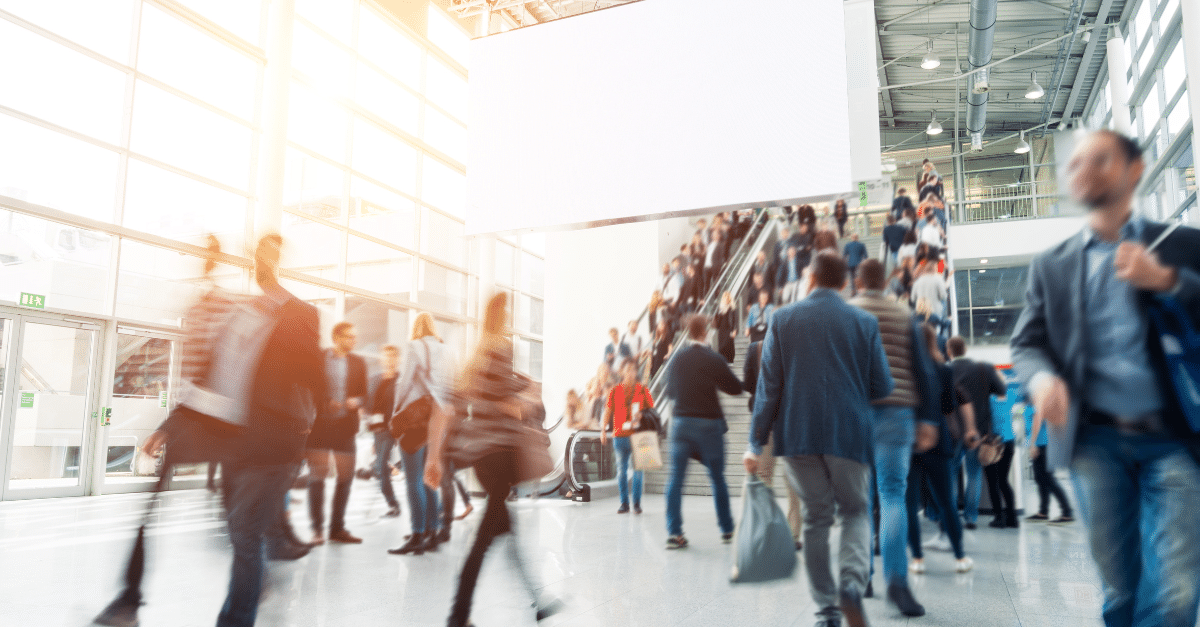
Applications in Real-Life Scenarios
The versatility of random sampling finds its application in various practical research scenarios:
- Opinion Polls and Surveys: Political polls, market research, and social studies often utilize random sampling to gather opinions and viewpoints from diverse populations.
- Quality Control: Industries employ random sampling techniques to ensure products meet quality standards by examining random samples from production batches.
Challenges and Considerations
While random sampling provides robust advantages, it’s not without its challenges, which proves the significance of collaborating with a partner like InnovateMR, who sources from a multitude of channels to diversify and collect the sample and ensure all cohorts are represented. A challenge without a trusted partner involves:
- Sampling Bias: Despite its unbiased nature, random sampling can encounter biases, especially if certain groups are inadvertently excluded or underrepresented due to limitations in the sampling process. Partnering with someone like InnovateMR who has a diverse recruitment approach helps mitigate sampling bias by ensuring representation across all cohorts, resulting in more accurate and reliable research findings.
Best Practices for Effective Random Sampling
Ensuring the reliability of statistical analyses through random sampling involves:
- Sample Size Determination: Determining an appropriate sample size is crucial to achieve statistically significant results.
- Randomization Techniques: Employing effective randomization methods to minimize bias and ensure fairness in sample selection.
The Indispensable Role of Random Sampling
Random sampling stands as a pillar in statistical analysis. Its ability to generate representative samples while minimizing bias has made it an indispensable technique for researchers, statisticians, and decision-makers across various industries and disciplines. Understanding the principles and best practices of random sampling is vital for drawing accurate and reliable conclusions from data.
About InnovateMR – InnovateMR is a full-service sampling and ResTech company that delivers faster, quality insights from business and consumer audiences utilizing cutting-edge technologies to support agile research. As industry pioneers, InnovateMR provides world-class end-to-end survey programming, targeted international sampling, qualitative and quantitative insights, and customized consultation services to support informed, data-driven strategies, and identify growth opportunities. Known for their celebrated status in customer service and results, InnovateMR combines boutique-level service with extensive global reach to achieve partner success.